Why you need to know the limits of your underwriting models
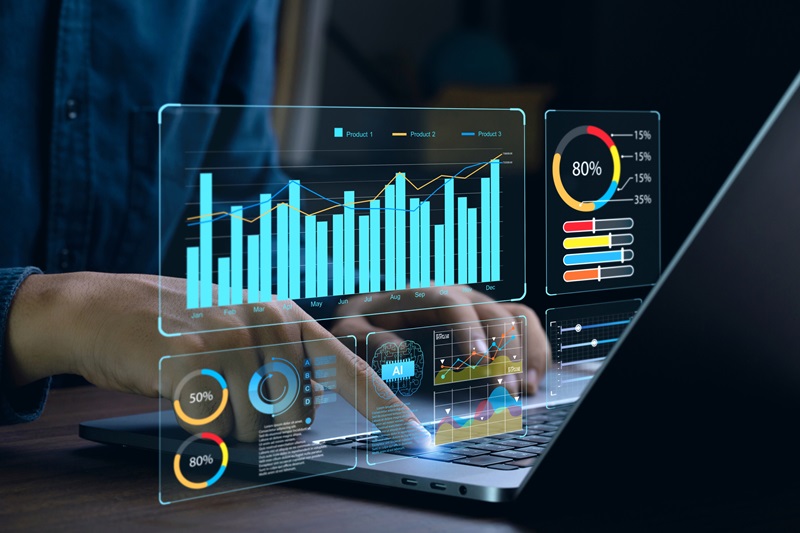
Vendors of underwriting models need to be transparent about the models’ limitations so underwriters and actuaries can understand where these limits could come into play, a speaker said last week at the CatIQ Connect conference in Toronto.
Every single data set for perils like flood, wildfire and severe convective storm has its limitations, said Carl Lussier, assistant vice president, personal lines – analytics, data, innovation with Definity Financial Corporation. Not considering these dataset limitations means the underwriter will miss an opportunity for flexibility when deploying an offer.
“Data isn’t only as good as the way it was collected,” Lussier said during the Underwriting Spotlight panel discussion. “It actually has a limitation to both the process [in which] it was collected and how it’s being used.
“And so, as an actuary and people that are going to be using the information for underwriting, if we are not paying attention to those limits, then we run the risk of actually deploying a solution which is clearly going to be wrong on average.”
Lussier used the example of a meeting a few months ago during which a reinsurer told him, “the following events are only considered as good as they are in the dataset there.”
Lussier’s response? “’Have you considered what’s going to happen to those events going forward, as we are seeing the climate is changing and a certain probability of loss for the past 10 years is not the same going forward for 15 years?’ And that was not considered. ‘So, you mean to tell me that the mean estimate and the variability of your estimate is actually going to be expanding both ways?’ Alright, that’s not a great story.
“But at the very least, now we’ve had a conversation and we can say, ‘This is the limit that we have and we can understand where that limit comes into play.’”
Another example is a water retention pond that’s classified as a lake, complicating underwriting pricing and efforts. Lussier said a few months ago he ‘had to take a walk’ with a broker in Ontario who was disappointed in the ‘punitive’ pricing and underwriting for the municipality he was in.
“The fact is that a lot of the features that I was using in the model to get the pricing and the underwriting were features that have been built by the city with international solutions, or artificial water retention ponds, which have now been classified as a lake.”
Accumulation of risk also comes into play, Lussier added, using the example of an accumulation of condo units in one building near a river.
Data collection and use also has evolved over the years, said another speaker, Jean-Raymond Kingsley, senior vice president and chief agent with Odyssey Re.
When Kingsley started in the industry in the mid-1980s, data collection was very costly. “The challenge I would say now is more how do you get through all of that data more than how do you collect more data, [so] you can do something effectively with it and you can actually make decisions.”
And data analytics has been stepped up over the years. “I always say to our stat that analytics is probably 50% of the answer, underwriting is the other 50[%],” Kingsley said. “But it used to be probably 10% analytics and 90[% underwriting] back in the early ’90s. Now, it’s probably a 50/50 share.”
Feature image by iStock.com/pcess609