Keys to using artificial Intelligence in the commercial insurance space
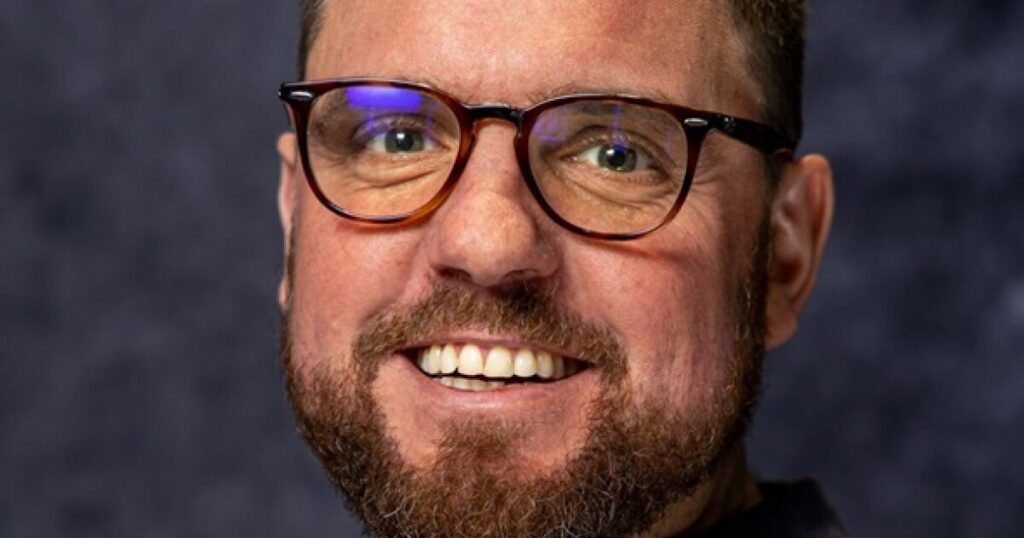
Transcription:
Transcripts are generated using a combination of speech recognition software and human transcribers, and may contain errors. Please check the corresponding audio for the authoritative record.
Patti Harman (00:06):
Hello and welcome to the Dig In podcast. I’m your host Patti Harman, Editor-in-Chief of Digital Insurance. As the use of AI and gen AI becomes more mainstream, the opportunities for its use are only increasing, allowing carriers and brokers to manage vast amounts of data craft policies to address specific risks as well as create new products. Joining me today to discuss how carriers are using AI in the commercial insurance space is Matt McGrillis, CPO, and Co-founder of the In InsureTech Send. Thank you so much for joining us today, Matt.
Matt McGrillis (00:44):
Hey, Patti, thanks very much for inviting me. It’s a real pleasure to be on.
Patti Harman (00:48):
I’m really anxious to hear what you have to share with our listeners today. So AI is definitely one of the hot topics of conversation these days, and I thought we could start with what you’re seeing generally in the insurance industry when it comes to adopting ai, and then we can look at maybe at some more specific use cases. So to that end, what are you seeing and does anything surprise you at this point concerning the adoption of AI and insurance?
Matt McGrillis (01:19):
Yeah, well, SEND is all around, its focus is mainly on underwriting and the underwriting of really complex P&C large commercial specialty risks. And what we see around underwriting is still the kind of predominant use of unstructured data and the real love of email and Excel and PDFs and everything that goes with that. And when someone tries to get a home insurance quote, then they put 10 pieces of information in and it gets a quote back. Obviously you’re dealing with that, but maybe a spreadsheet with a hundred thousand properties on it. And in order to process that quick, make sense of it quick, make the proper assessment of the risk and do all of the decision-making around that very quickly is very difficult. And that’s where we’re really seeing the need for potential use cases around AI. So it’s dealing with that unstructured data, trying to summarize it, make sense of that as quick as possible.
(02:28):
We’re then seeing AI, which is getting better and better, especially the large language models around the kind of comprehension. And it can say, well, is this risk in my underwriting guidelines? Is everything fine? It then goes into pricing where they also might be using AI around all of that, around both on the actuarial side and the data science as well. And obviously then when it goes post underwriting around claims management, does this claim fit the policy and fraud detection, that kind of stuff. I mean, there’s more and more uses of this all the time. I guess I’m not surprised by the fact that there’s been relative slow adoption in terms of everyone. There’s a real FOMO about it all really. Everyone is desperately thinking, we need to get on with this, we need to get on with this. And very few are making practical, really good uses of it at the moment because I think they really, underwriters and naturally, especially underwriting organizations and actually risk averse, they need to figure out actually what does this thing mean? What does Gen AI mean to me and my company? And how do I need to protect myself and what policies do I need to get in place? So there’s been a lot of that going on, and now a lot of internal teams within carriers are now saying, right, well now we’ve done that bit or done some thinking, or maybe we feel as though we’ve fallen behind because we haven’t done enough. They’re now really trying to get into it in whatever ways they can.
Patti Harman (04:08):
Well, I think part of the challenge too is that the technology is changing so quickly. So as soon as you think you’ve gotten your arms around it, it’s like, well, it’s changed and there’s another iteration of it and it can do more than the previous versions did. And so for carriers and for underwriters, since you’re right, they’re the ones that kind of look at the risks for different companies and how they’re using this. So I can understand that they would be a little bit more cautious maybe about the implementation aspect of it because they’re trying to look at it from so many different angles. So that’s pretty amazing. As both carriers and government entities try to regulate and set standards for the implementation of use of AI, what are some of the factors that need to be considered and are there certain areas where you think they should be focused as they develop standards and regulations for its use? And I realize that this is kind of a general question, but I’m thinking in terms of healthcare, the legal industry, that sort of thing, there might be certain areas where whoever’s setting standards or regulations is going to have to pay some serious attention to what they’re thinking in long-term ramifications of those decisions.
Matt McGrillis (05:23):
Yeah, absolutely. And people are only really thinking because it’s so in its infancy. People are only thinking now about, actually we hadn’t considered that and now what do we do about this? And now what do we do about that? And you heard obviously open AI’s new model was released and a lot of people, there was a lot of stuff in the press about its biohazard risk rating going from low to medium. I mean, I guess the tip of the iceberg, there’s going to be so much around that. I mean, obviously everyone’s also gotten lots in the press about copyright and intellectual property, about how have these models learned how they can do all of these things and answer all these questions, but how did they know? And yeah, people, it’s very hard.
(06:17):
I’m sure there’s going to be lots of lawsuits to come in future about people as they get more and more into that. I mean, from a company perspective and the use of these models, it’s really around, well, what if you get or are putting the wrong kind of data in there, things like maybe personal data or anything to do with identification of individuals. It then comes in all of the data policies like GDPR and that kind of things come into play, the right to be forgotten. How does that even work in large language models? It’s very hard. No one really knows at the moment and can you really unteach a large language model if you ask it to? It’s very hard. And then there’s lots of stuff as well around the kind of fairness and bias as these large language models are learned. They’re learning on material that might have bias. And that’s very hard as well for companies to be able to span that and truly understand what they need to do around it.
Patti Harman (07:33):
I don’t think people realize how complicated part of this is. And when you were talking earlier about unstructured data, the fact that you can even take that information and collect it and put it into something that could be used to assess risk or to underwrite large losses or that sort of thing. To me that is absolutely phenomenal that we have arrived at that point because one of the things that I covered for a while was the claims industry. And when you look at an insurance claim, there are just so many parts and pieces to all of that. So the ability to be able to take a lot of that information from so many different sources and put it in a far more structured format really is going to be huge for this industry. So do you think the private industry will be able to establish standards and best practices maybe more easily than government entities when it comes to managing how AI is used and what are some of the challenges for each group?
Matt McGrillis (08:39):
I guess companies are very likely to be able to do it quicker. The problem is, will they align with what the government’s thinking as well? Inevitably not. And yeah, there’s going to be a lot around that. I mean, I think there’s so much to consider, especially when you think of the jurisdictions around use where the economy and the use of AI and businesses dealing with global customers in areas where the regulations about AI may potentially differ. So the kind of state to state in the U.S. or Europe. And yeah, it is going to be really, really complex. And I think businesses just need to be, I guess, prepared to pivot very quickly as these new regulations come in. And I’m sure, well, I think everyone agrees there is a need for it, but it’s just how it’s going to work. Who knows? But yeah, everyone’s watching with bated breath.
Patti Harman (09:50):
Well, I think it’ll be a challenge. In a previous life, I was involved in developing industry standards and I remember how long it would take to create those. So I can only imagine what will be involved in trying to regulate some aspects of AI because you’re right, you’re looking at it across multiple industries and jurisdictions and countries, and everybody has different regulations that they’re already operating under. So it will be interesting to see what happens. You mentioned earlier about AI being used in personal lines, and I know that it’s used to gather records for underwriting and life, property and auto insurance. Are you seeing a greater adoption in some commercial lines as well, and how is it being used? And then if there is adoption, are there certain lines where it’s almost easier to introduce the use of AI?
Matt McGrillis (10:47):
Yeah, I think that it tends to vary. There’s the common problems where it’s going to apply across all lines. So things like renewals where you’ve got a load of unstructured data, so paperwork, attachments, emails maybe that have come through, they came through last year, they’re coming through again this year. What’s changed? Has anything changed? There is vast amount of data to validate, and I think that we are definitely looking at that as a real area where AI can really help. So renewals and the summarizing of information, the summarizing of changes of data. So I think that that is really going to be, it’s a common problem. Underwriting rules and validations, that is again, something that will cover all of the different business lines. They all will have their own guidelines and they all want to do the assessment of risk and the assessment might come in structured or the risk data might come in structured or unstructured form and they use AI or can use AI to do all of that, obviously, where you are getting huge amounts of data that’s coming through then yes, property, and you can have a look at all of that, the schedules that are coming through, validating if there’s anything that might be outside of risk appetite in amongst the needle, in the haystack, amongst all of the different properties that might be coming through for things like E&O.
(12:33):
Whereas before you were looking at data, there were data sources available to see have there been any kind of negative publicity about X, Y and Z? Well, that’s another, people are asking large language models to summarize that kind of thing. Obviously there’s dangers because of that, it’s not always going to get it right. But yeah, that’s the areas where we’re seeing lots and lots of movement, lots and lots of startup InsurTechs starting around that space. And it’s, well, something that we’ve been doing for the last five years before the Gen AI came out, but obviously now there is more and more opportunity to use Gen AI for that kind of thing. And it’s taking up a lot of our time and product budget percentages investigating and really trying stuff out in that space.
Patti Harman (13:33):
Yes. I’m sure there are a lot of interesting opportunities that it presents as well for you guys. So this is a great topic. We’re going to take a short break and we’ll be back in just a few seconds…Welcome back to the DigIn podcast. We are chatting with Matt McGrillis, CPO and Co-founder of Send, about the use of AI in the commercial insurance space. And Matt, we were talking a little bit before, but I was wondering, do you have any concerns as companies adopt or integrate AI into their business practices? And are there certain things that they should be watching for… factors or pitfalls that they should at least be aware of as part of their implementation?
Matt McGrillis (14:20):
Well, I guess, yeah, especially looking at Gen AI, we all know it can get things wrong sometimes, and I guess we’ve all seen the disclaimers on Google and everywhere where it’s using the answers may not be correct, and that really needs to be factored in to business processes as well and where the data is stored. Obviously, everyone was really worried when they thought that there was the monolithic huge models that are being built up that how are we going to separate our things have come along more and more thought of being around segregating the data down to a certain client, which is, it helps, but it makes you think that the design patterns around the technical implementation of these are not fully mature. My background is I’m a techie, I’m an ex developer. Things are just changing so rapidly. The design patterns that you might have around the traditional technologies are in their infancy.
(15:35):
And what it means is that there’s lots of, I say trial and error, there is some trial and error in terms about how you can integrate those solutions in, but there’s also the fact that things are moving so quickly that even we’re seeing in it that when the GPT four was released, we were like, this is incredible. We jump on this for all of these different use cases that we’ve been holding off for a while until something like this came along. And then even between GPT-4 and what’s there now, there’s so much extra, which means we’re having to do a lot of rework of the stuff because actually you don’t need to do it like that anymore because it’s improved and you could do it in a different way. And you’ve got to factor in that it is exploding in terms of the functionality. Not only its abilities are changing, but how you are interacting with those models are changing too. And you need to be aware that if you’re going to delve into the world of integration of the large language models, you need to be aware that it’s not a once and done, you’re going to have to keep on top of these things. And yeah, that’s a real lesson. I say a lesson learned. We knew that we would have to do it, but we didn’t realize the speed of the iterations of the models that are coming out. It’s just incredible.
Patti Harman (17:10):
Yeah, it really is pretty amazing. I know for Digital Insurance from a coverage perspective, I feel like we’re always trying to get our arms around what’s coming out and what should we be watching and what’s on the horizon. And I was joking with somebody, I used to ask people, oh, so what do you see in the next three to five years? And now it’s like, so what do you see in the next 12 to 18 months because it’s changing so quickly now. And talking about that change, what excites you the most about how AI is transforming the insurance industry? Are there areas where in the commercial space you see that adoption will have a really positive impact?
Matt McGrillis (17:53):
I think yeah, I mean absolutely. As the models evolve and they change, it just opens up so many new use cases. Just I think, was it last Friday where the new open AI models came through and the cognitive models with Humanlike reasoning. I had a terrible night’s sleep on Friday just because my head was just buzzing about, wow, this is going to, we’ll be able to do this. But also just it opens up use cases in so many different areas. It wasn’t very good at math in previous iterations. It made the press, I mean, it is so good at so many different things, but there were simple things like it was getting things wrong, like counting the amount of “I”s in certain words or whatever to then watching the videos of it building out complex mathematical expressions. It was just mind blowing. It was really, really kind of exciting to see.
(19:06):
But also as that is changing and being able to, there were so many limitations in terms of you could feed it spreadsheets and ask it questions. And like I say, underwriters, who live in spreadsheets and the questions that you were asking of it before were often wrong. The answers were wrong, but this changes a lot of that kind of stuff as well. So it’s really exciting to see that. Exciting to see the different use cases coming through. And how I say underwriters especially can make use of that. It’s the kind of art and the science bit. And whereas underwriters were traditionally burdened with a lots of admin work now you can get a lot of that done. I watched a video this morning around the use of pivoting slightly the use of AI in law firms. And I did think when it first came out and hearing that it’s now getting amazing marks in US bar exams and thinking, goodness, lawyers are going to be out of a job.
(20:26):
But lawyers are seeing this in exactly the same way that underwriters should see this, that actually all of the boring cut and paste, the comparisons, all of that kind of stuff is now going to be helped a lot by Gen AI. And I think they’re looking at it in the same light as underwriters when it allows them to do what lawyers do – the art of law. It allows underwriters to do the art of underwriting, and I think that’s really exciting. It just means that things will go on and on and on. People will be a lot more effective. Doctors and people in the health industry are going to be a lot more effective. I mean, yeah, it’s exciting.
Patti Harman (21:12):
Yeah, it allows you to see the anomalies and know where to focus your attention on and so that you’re not spending time on things that maybe may not matter as much to underwriting or your example in medicine, doctors can focus on certain things and it’ll flag and say, Hey, this requires a little bit more investigation or a little bit more testing or whatever it may be. So the insurance industry has long had a reputation for not being a leader in the technology space. And yet InsurTech is constantly evolving, as we’ve said, to meet new needs and allow carriers to create new products. How important is it for carriers and agents and brokers to be agile in their approach to technology? And what are some of the greatest benefits to having a flexible approach to a technology adoption?
Matt McGrillis (22:09):
Yeah, I think a lot of people, especially in dealing with insurers that are dealing with complex risk, they typically have policy admin systems that have been in place that are basically populating and being the single source of truth for risk. Those policy admin systems are often fairly old. They are the backbone of their organization, and those organizations are therefore terrified of touching those. They’re often because they tend to be older, tend to be based on old technology, which is not so agile and will inhibit the use of that. And this is seeing, this is where Send was, I guess born to really sit on top of those policy admin systems and be the agile platform that allows underwriters to leave their policy admin system in place, but actually be the single source of truth for all of their data, the single pane of glass where they can have a look at all of the premiums and claims and all of their underwriting workflows. And I think as I said before, because things are evolving so fast, then underwriters or carriers are really looking to work out how they’re going to, what systems, what applications are they going to put in place to enable that real agile way of working, that ability to be nimble, the ability to try things and maybe think, oh, that didn’t work, and then try something else and basically experiment and move quickly. I think that’s what it’s all about.
(24:09):
This kind of revolution that is just about to happen is going to change everything, and that is just people need to be on top of it.
Patti Harman (24:24):
Yes. I totally agree. I know even within my editorial team, we cover AI and what’s going on, and by the same token, I’m encouraging them to experiment with it. How does it work, what can it do? And I know several of the programs that we use to write, all of a sudden AI is popping up and saying, oh, do you want to say this this way? Most of the time I’m still saying, no, that’s not what I want to say. But that ability is there, and now it says, so do you want to write with AI? And I’m thinking, yeah, no, but I think as it evolves, it’ll be really exciting to see what happens going forward.
Matt McGrillis (25:03):
Yeah, it’ll be really interesting to see humans the way that they interact with it in a kind of B2C way, is a customer ever going to want to speak to an AI where they always want the personal touch. It’s just like it’s really evolving, isn’t it?
Patti Harman (25:22):
Yes, it definitely is. So we’ve covered a lot in the last couple of minutes. Is there anything that I haven’t asked you about adopting AI for commercial lines that you think our listeners should know, or anything else I didn’t ask that you’re like, you guys really need to be watching this or aware of it?
Matt McGrillis (25:40):
No, I mean, I think we’ve covered so much. It’s been a really great conversation, but I mean, I think insurance aside, it’s like an exciting time. I was kind of thinking when I was watching all of these new evolutions in AI and their models and just all of the sci-fi stuff that we’ve probably watched as kids, whether we were watching Hal 9,000 in Space Odyssey or Jarvis in Iron Man, and we are thinking, oh my goodness, this is actually starting to happen now. So I mean, it is terrifying and very exciting at the same time. So the potentials for it are so huge. The threats are so huge. I mean, everything will change because of this. It’s like I just see it as big as before the internet and now the internet is going to be, we’re going to go through a similar shift. So I guess everyone just needs to be thoughtful about it and be ready for it and be on top of it.
Patti Harman (26:51):
Great. Thank you so much, Matt for sharing your insights with our audience. Thank you for listening to the Dig In podcast. I produced this episode with audio production by Adnan Khan. Special thanks this week to Matt McGrillis of Send for joining us. Please rate us, review us, and subscribe to our content@wwwdigin.com slash subscribe From Digital Insurance, I’m Patti Harman, and thank you for listening.