How Wells Fargo is deploying the White House's AI Bill of Rights
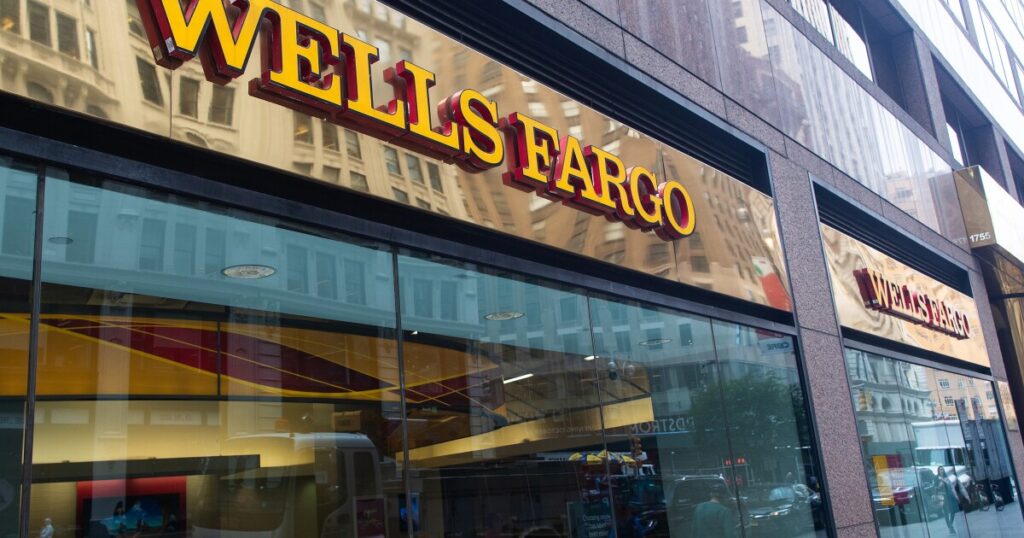
Late last year, the White House issued a blueprint for an AI Bill of Rights in October as a guide to companies like banks that use artificial intelligence. It laid out five rights consumers should have as companies deploy AI: protection from unsafe or ineffective systems; no discrimination by algorithms; data privacy; notification when algorithmic systems are being used; the ability to opt out; and access to customer service provided by human beings.
“It seems like every day we read another study or hear from another person whose rights have been violated by these technologies,” said Sorelle Friedler, assistant director for data and democracy in the White House Office of Science and Technology Policy, said at a December Brookings Institution event. “More and more we’re seeing these technologies drive real harms, harms that run counter to our core democratic values, including the fundamental right to privacy, freedom from discrimination, and our basic dignity.”
Banks like Wells Fargo have to take such government-issued warnings seriously. They use AI in many places, including customer service, cybersecurity, marketing, lending and fraud detection, and they work with consumers — in Wells’ case, 70 million of them.
“The idea that you can replace humans in the loop, where there are no human beings anywhere intervening in the flow, I don’t think it’s going to happen,” says Chintan Mehta, CIO of digital, innovation and strategy at Wells Fargo. “And I don’t think it should happen.”
Chintan Mehta, CIO of strategy, digital and innovation, has been helping Wells Fargo implement the bill of rights. His team also partners with Stanford University to test its research on human-centered AI. In an interview, Mehta gave his take on the White House’s recommendations and what Wells Fargo is doing with them.
What struck you about the AI Bill of Rights when you first saw it? Where do you think it would have the biggest impact in a bank?
CHINTAN MEHTA: My personal opinion is it’s the privacy piece as well as the human heuristic layer at the end of it, which is a fallback ecosystem.
The idea that you can replace humans in the loop, where there are no human beings anywhere intervening in the flow, I don’t think it’s going to happen. And I don’t think it should happen, to be very clear. That is going to have a profound impact on choices you make around how you design products, how you safeguard customer offerings.
Is this as simple as always having a ‘tap here to talk to a human’ button? Or is it more complicated or more difficult than that?
My hunch is it has to be more nuanced, because what would allow a customer to know that, hey, look, I should have a human here because I’m not comfortable with what is happening? I think a lot of the times when something goes not according to the intent, it’s not because somebody’s intending to do something wrong, it’s just that nobody noticed it. It is important that we think of the ways in which potential downsides can occur with an algorithmic deployment, and then have backup plans for each step along the way. That could mean, as an example, in lending the AI might give you a risk scoring for something, but then at the end of the day, a human being is going to read the risk scoring, they’re also going to check documents themselves. They’re going to add a layer of manual scrutiny on top of it, and then say, fine, let’s go do it. And then obviously there’s another example that you’re describing, which is the moment the customer feels very uncomfortable with how this is shaping up, they can say, look, I want to go talk to a person.
So you’re never just going to send an AI engine out there by itself to make decisions. There’s always going to be somebody monitoring and checking it.
I can’t say that that will never, ever happen in the future anywhere in the world. But as of now, I don’t think that’s going to happen.
Some AI chatbots are trained to perceive when it’s time to refer an interaction to a human, for instance if a customer seems to be getting angry or frustrated.
One thing we do programmatically is if the same intent and entity show up in two or three turns — you typed something, I gave a response, you typed again something which was very similar to that — our response at that point on the second turn or third turn will include, “We are not able to understand, would you like to talk to somebody?”
What are some of the data privacy concerns that might affect banks?
One is the right to forget, which is, generally speaking, not only do I want my data not to be shared, but being able to say “don’t use my data in your model” is a very effective way of making sure that people have choice when it comes to what algorithms are going to do with what you would, in an ideal sense, call your digital fingerprint.
Is it difficult to make sure your AI training data doesn’t include data from people who have opted out?
Some of these have hard operational challenges. But what I was thinking was, let’s say you are a customer today. You are comfortable with your data being in the models being built. Three months later you come back and say, no, my data shouldn’t be part of the model. Now are we saying that it’s applicable retroactively, or is it on a go-forward perspective? Because if it’s retroactive, then that model has already learned stuff, now you have to figure out how to deal with that. Now compound this by a million people in a day. We have 70 million plus customers, and if a million people are opting in and out constantly, what does that mean in terms of the model’s ability to actually remember things? Because large language models by definition have to remember the text they have been trained on. So that is one operational challenge of it.
The first principle of the AI Bill of Rights is, “You should be protected from unsafe and ineffective systems.” What does this mean to you?
Is it resilient? Is it reliable? If a lending system is built on AI, is that system resilient enough that it’s going to be available? Is it going to actually perform as fast as it needs to perform, and is it going to actually do the thing it’s meant to do? Safety and effectiveness is a function of what you would expect of any digital system: Is it available? Is it responsive? Is it actually meeting the intent it was built for? A system that is not available is never going to meet its purpose and it’s not going to be safe because it’s not doing what it’s supposed to do.
What about the second principle, “You should not face discrimination by algorithms and systems should be used and designed in an equitable way.”
This is about algorithmic bias — data bias as well as whether the algorithm is going to skew towards certain types of data. If your data to begin with already had a predisposed skew of a certain kind, the algorithm is just going to amplify it. So how do you make sure that the dataset and the algorithm has the ability to detect that?
I think the bill of rights document talked about making sure you have diverse developer teams. Is that the kind of thing that you think can help? And what other efforts might help?
I think having diverse developer teams is relevant. There is also a separation of duties component. So the way we do it at Wells Fargo, as an example, is the team that develops the models is not part of the group that does the review of the models. They are a bunch of data scientists as well. It’s time consuming, but it’s the right thing to do, which is to say, where did you source this data? Why did you use this data? What model did you use? Why did you use this model? What is the output you’re getting? They recreate the whole thing independently.
Then there is a component of, okay, who takes it into production and the experience, and that’s a separate team. Building a model is one thing, but bringing it to life in an experience is another thing. So there’s a production group that takes care of, how does it fit into the experience? Does it make sense to launch it? Even when we launch it, we launch it with a champion/challenger structure, which means that initially we’ll expose it to about 0.1% of the population. We will monitor it. And then if it’s doing what it’s supposed to do, as in it’s safe and effective, then we slowly ramp up.
For things like lending and hiring, you probably have to test the outcomes, right? Who was approved and who was declined, or hired or not hired, to see if the system was really being fair or if there’s any kind of weird bias.
There are two things we do. One is we do back testing, which is essentially decisions that we have already made. The second is, the independent model risk governance team at Wells Fargo I mentioned before has built a tool that allows us to frame the attributes and signals that led to that adverse decision in any given model. It’s the explainability component of the model where they actually spit out a significant amount of detail that says that, in this example, for this record, here’s why this model reached this conclusion. It was because of these signals, which then can be checked by people to say that, look, that signal shouldn’t be given that much of a weight.
The fourth basic principle of the AI Bill of Rights is, “You should know that an automated system is being used and understand how and why it contributes to outcomes that impact you.” Is that something that banks are pretty good at today, or do you think there need to be alerts to consumers that their data is being used in marketing or customer segmentation software or what have you?
Two things have to happen. When you’re taking the data for training, you have to be very clear that this data’s going to get used for this setup. And you have to be very clear about where this data that we have is going to get used, for fraud models, for personalization, for marketing, whatever those things are. Most banks are reasonably decent at describing what they are going to use it for when it’s transactional. So meaning, I can use your address and so on and so forth for managing fraud, because I don’t want you to be subject to scams. Where we need to get better and we are getting better, I think, collectively, is when data is used to generate a marketing offer or a next best action. Do I tell you that this was generated by AI? Most of the time today it is clearly articulated that it is a personalization offer or it’s a next best offer, which usually people implicitly connect to coming from an engine. But I think that could be more about disclosing it, saying, it’s this kind of an AI engine versus that kind of an AI engine.
I don’t remember ever seeing a disclosure like that, but maybe it’s been in small print.
If you log into the Wells Fargo app, just below the account summary, you will see those offers. And above that it basically says it’s generated by our automated marketing process. Now, I think the question that you’re asking is, does that make it obvious enough for the customer that this is coming from an AI? But I think the differentiation is that AI is going to be everywhere. So do we call out AI there or do we call out which process created that?
We already talked about the fifth principle, which is around always having a human in the loop. Can you tell us about your work with Stanford University’s Human-Centered Artificial Intelligence research group?
Stanford’s Human-Centered AI was set up in late 2019. It’s a cross-discipline group between the humanities, engineering school and the business school. And their primary research vector is to make AI equitable in terms of where it is deployed and who can use it, but then at the same time also make it humanized, where it doesn’t go off on tangents that it’s not supposed to go. So how do you safely use it while you’re innovating in that context? We have a resident scholar who works at Stanford evaluating some of these things. When a paper is published, we try to actually implement it and then feed that back into the process so that the academic rigor is not just purely academic, it’s also practical.