How insurers can leverage generative AI to bolster risk management
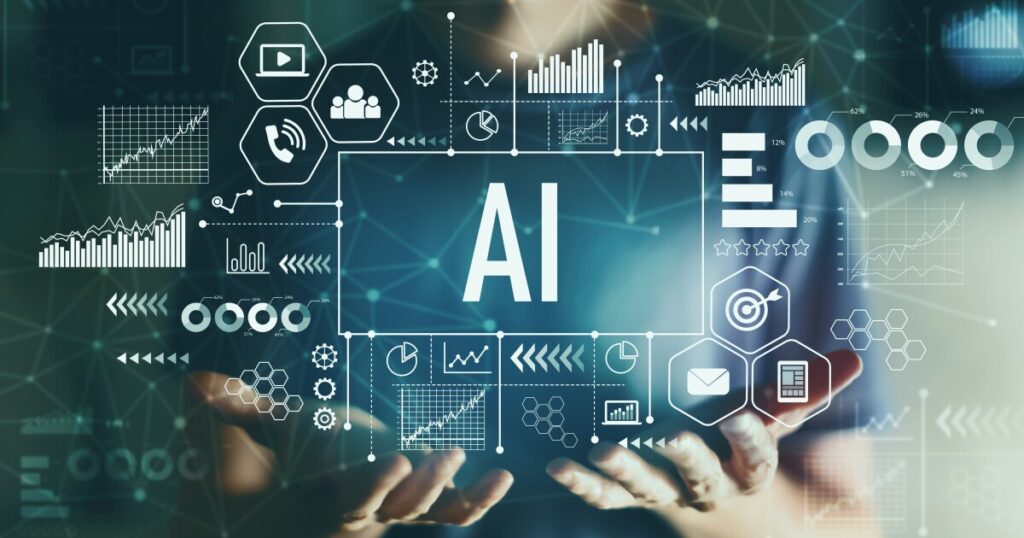
Second in a series on how the insurance industry now sees Gen AI and its potential.
Insurers applying Gen AI to risk management are finding a variety of relevant applications of the technology for that purpose, according to industry professionals and observers.
Jessica Groopman, senior innovation advisor, Intentional Futures
Christophe Testi chris@creatives
Risk mitigation has many facets that are ripe for Gen AI, according to Jessica Groopman, senior innovation advisor at Intentional Futures, a digital strategy and innovation consultancy. “That includes risk profiling, analyzing huge amounts of data and identifying risks, and assessing underwriting eligibility or ineligibility, looking at regulatory compliance, and assisting in reporting or compliance monitoring,” she said.
Aside from identifying risks, Gen AI can power risk mitigation, in the form of predictive maintenance, which is also used in other industries, Groopman added. This uses AI to analyze Internet of Things (IoT) devices and predict equipment failures, which can then recommend specific steps that will reduce claims (and therefore reduce risk).
Similarly, applying Gen AI within the context of parametric insurance can harness these technologies for reducing risks, Groopman explained. “Parametric insurance, enabling insurance access to developing markets, or for previously uninsurable risks, can use sensor data, real world data, to cover insurance, or at least to preempt claim processing around areas like natural disasters or increasing risk and resilience issues in agriculture, in food security, and in the energy sector,” she said.
Kimberly Harris-Ferrante, distinguished vice president analyst, Gartner.
The amount of risk data that insurers must sort and analyze presents a challenge for being able to use that data for risk management, stated Kimberly Harris-Ferrante, distinguished vice president analyst, Gartner.
“Is it going to be too overwhelming for a human to look at? Is it going to slow down or clog the system,” she said. “We hear a lot about knowledge transfer, how do I bring my newer, less experienced underwriters up to speed from the experience of those who might be aging and leaving the job force.”
Lauren Finnis, head of commercial lines, insurance consulting and technology division, North America, Willis Towers Watson
Insurers working on risk management frameworks can take practical steps for assessment using AI, according to Lauren Finnis, head of commercial lines, insurance consulting and technology division, North America at Willis Towers Watson (WTW). “Once you’ve got your committee together that’s really assessed your risk and your opportunity and how you might use AI,” she said, “then [it] comes to educating your workforce and putting your appropriate guardrails on, by doing the work to teach your workforce how AI could benefit them and do it in a governed way.”
Nationwide finds that corporate and operational risks are the biggest risk concerns to manage, according to Todd Lukens, senior vice president and chief technology and information security officer at the carrier. For underwriting actuarial functions, the firm either generates its own models or partners with third parties.
Todd Lukens, senior vice president and chief technology and information security officer, Nationwide.
“When we think about the usage of AI, Gen AI specifically within the context of Nationwide enterprise, it really is making sure that whatever data we feed it, whether that’s internal intellectual property or otherwise, has the appropriate protections up to and including, how do we anonymize that data?” Lukens said. “How do you make sure that before we release that data into the model, we’ve removed all of the sensitive data elements? That’s all part of what we’ve been calling our AI data operations. We think about the data pipeline and how it gets fed into these different AI capabilities.”