Getting to a nondiscriminatory outcome with AI
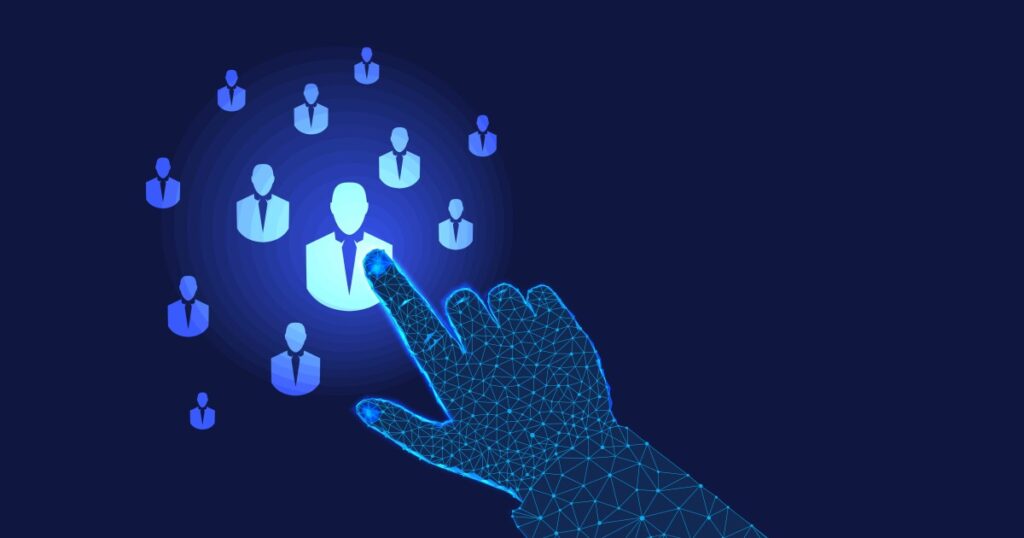
Proponents of the use of artificial intelligence trumpet the fact that a machine left to its own devices is able to make unbiased decisions and prevent fair lending violations.
Yet, a concept common to computer science from its earliest days is “garbage in, garbage out.” In other words, the underlying data used for a system to generate a decision could be responsible for perpetuating a fair lending bias because that underlying data was the result of past biased decisions.
“Since so many of the generative and machine learning AI applications are based on consuming historical data, the risk of encoding bad data, redlining for example, is very real,” warned a paper from consulting firm BlackFin Group.
READ MORE
AI has a tendency to repeat the past. “You’re building up on a set of histories,” said Andrew Weiss, a partner at BlackFin who was one of the authors of the report.
He is a former Fannie Mae executive who ran the group that developed Desktop Underwriter, which is rules-based technology.
In making a decision, the lender needs to be able to justify why it was made, Weiss said.
“Using AI to do the traditional underwriting job may not really be a good idea,” he said. “On the other hand, using AI [for] part of what underwriters do, which is examine documents and make sure that those documents contain valid data, that’s something that isn’t really subject to the same kind of judgment.”
Artificial intelligence has become a catch-all term for technologies that are capable of several different activities, and to some people, it’s like a “superpower,” said Tim Ray, CEO of VeriFast.
“There’s lots of nuance and edge cases and people are people; everyone has a story and everything’s not so black and white,” Ray pointed out. “So how do we use AI to help with those decisions?”
It only does what it is taught to do, he continued.
Agreeing with Ray about AI becoming a buzzword, “Almost everything is getting called AI these days,” Weiss commented. “People are relabeling old things that were invented before AI was ever really used.”
So far, only a few use cases for AI have been approved for real estate finance, said Mortgage Industry Standards Maintenance Organization President David Coleman. One is for code generation, and the other is for monitoring functions around documents.
“I haven’t talked to anyone yet that is looking at a production version of AI for decision making,” Coleman said. What has come out in MISMO workshops on the topic is that the industry wants more discussion about it, as well as the development of a taxonomy and a vocabulary.
He noted the government already has put out a dictionary with approximately 517 terms.
“We’re not looking to recreate anything but what we want to do is make sure this is where the industry is in making the best decisions,” Coleman said.
Its best use case in the mortgage process may not be in making the decision, but getting to the point where a decision can be made, several people interviewed for this article noted.
If a person is not making the decision, in theory using AI should lead to better or more unbiased outcomes, said Subodha Kumar, the Paul R. Anderson Distinguished Chair Professor of Statistics, Operations, and Data Science at Temple University.
“We have to understand [that] biases not only come from data biases but also come from algorithms,” Kumar said.
Some people have proposed that AI algorithms in mortgage lending be designed to exclude race as a characteristic.
“The problem is that even if you don’t use race data, there are many other features that will be related to race,” as thus used as a proxy, Kumar pointed out, adding it is not a problem related to mortgage; it has been raised when it comes to facial recognition.
But one of the beautiful things about AI is that it can sort through so many variables that allow for a more holistic view of that data, Kumar said. That allows for the reduction of bias.
“But the most important thing is that there will need to be oversight,” Kumar warned. Manual oversight provides checks and balances on the statistical side.
An algorithmic fairness technique mentioned in a paper by the National Fair Housing Alliance and Fairplay AI is called distribution matching.
It is a technique that uses additional objectives or targets so the outcome for any one group closes resembles that of the control group, said Michael Akinwumi, the NHFA’s chief responsible AI officer.
As a result, the outcomes are also distributed fairly, “so that the risk of discrimination is minimized,” Akinwumi said.
“When [AI] is used responsibly, it can actually expand the credit box for consumers,” he said, giving them more opportunities to obtain housing, both purchase and rental.
If the algorithms are trained to regard disparities in lending outcomes as another form of error, mortgage approval rates for Black and Hispanic homebuyers can be increased by between 5% to 13%, this study found.
As the Townstone Financial case proves — even though so far it has been a loss so far for the Consumer Financial Protection Bureau — fair lending enforcement actions aren’t limited to lending decisions, but also around marketing and customer contact.
Last September, the CFPB issued AI use guidance for credit denials, pointing out the technology is not a get out of jail free card when giving consumers the reasons why they did not get their desired product.
“Technology marketed as artificial intelligence is expanding the data used for lending decisions, and also growing the list of potential reasons for why credit is denied,” said CFPB Director Rohit Chopra, in a press release. “Creditors must be able to specifically explain their reasons for denial. There is no special exemption for artificial intelligence.”
In other words, using a complex algorithm does not abrogate the lender from its responsibility under the Equal Credit Opportunities Act.
“Where we really think it matters most is getting people off the phone, it’s getting people out of email, and letting them do their job,” said Hoyt Mann, president of Alanna.ai, which works with the title insurance industry.
Society wants instant 24/7 access to the information that they need, and AI is perfect for being inserted into the communication chain, Mann said.
But Mann has a strong opinion on one important aspect of AI usage: “We have to keep humans in the loop.
“Our work is going to change, but humans have to stay in the loop,” Mann continued. “There’s a risk for utilizing AI in different parts of your business and understanding the levels of risk is important.”
Using it for communication has a lower level of risk, as long as the lender is not giving out information that changes the course of the transaction, Mann said.
The Townstone case hinged on statements made over time on a radio program that the CFPB said discouraged a protected class from applying for a mortgage.
It comes back to the training data used for the AI system, Mann said, as well as how recent that information is, and importantly, how the users ask the question of the system.
When people ask questions of each other, it is in a context predefined by their roles.
“AI doesn’t necessarily have that unless you set it up,” Mann said. “People ask ambiguous things all the time, and without that context, the answers can be a little bit wonky.”
That is why users have to verify the answers AI gives. If anything, when giving an erroneous response, AI has been known to double down, so it is pertinent to ask where it got its answer from, Mann said.
Microsoft’s product is called Copilot; Mann termed that the perfect name, because “where [AI] needs to be is in the passenger seat. Not in the driver’s seat.”
Vishrut Malhotra, the CEO of Rexera, previously worked at large investment management firms on Wall Street, which would use quantitative algorithmic models to trade.
Those firms understand “that humans have conscious and subconscious biases, and those lead to poor decision making, which lead to poor performance in portfolios,” Malhotra said. So algorithms were created that could remove those biases for the most part were effective at that task.
“So I think there’s a lot we can learn from that industry in terms of how do we test? How do we look for bias? How do we look for poor decision making in these models?” Malhotra said “I think those ideas carry over even in the new world of AI.”
Step one is checking your data, Malhotra said, the next is to understand what the model does. Importantly, the model should not be a black box.
For any AI application the user has a complex number of steps or a complex workflow. “What we do is we break it up into smaller pieces and we ask each AI model to perform one small piece so that it doesn’t become this large black box model for us,” Malhotra said. “It’s easier to understand the decision making of a model if the steps are smaller.”
Rexera next pushes to the models to provide a detailed chain of thought, explaining how it came to the decision, so it can further mitigate the black box issue.
An approach to take for mitigation is to create an alternative model and use that as the check.
“Let’s say you have a model that makes some decisions and you are concerned that there may be bias in the data or within the model itself,” Malhotra said. “What you can do is create another model, whose job is to evaluate for bias and be sort of this bias detector.”
The second model can ask the first to convince it that no bias in the decision making existed.
Agreeing with Mann, Malhotra said the best way to think about these models right now is if they were interns, someone who needs the right oversight, training and support to perform their tasks.
An AI application that Rexera offers to clients is to evaluate if a loan could be eligible for purchase by Fannie Mae or Freddie Mac. But it does not decide if that loan will be approved. It is a low risk use case because Rexera is relying on quantitative data sets, Malhotra said.
Fannie Mae and Freddie Mac have some fairly complicated underwriting rules. Rexera trains the AI model to check for those rules.
But after the loan is run, a report is generated and the operations analysts look at the file. If they disagree with the outcome, the parameters of the model can be changed.
It is not a process that, as one classic infomercial for a rotisserie oven claimed, “set it and forget it.”
Humans still need to have a role in these complex use cases, Malhotra said.
He pointed out that Wall Street firms have a strong compliance culture and the mortgage industry would need to adopt that mentality. The compliance teams have to step up when it comes to using AI, by giving lenders guidelines and then be shown that those are being followed.
The “happy path” in mortgage lending is someone who is a W-2 employee and makes over $100,000 a year of income, Ray said.
“That’s the easy thing to demo and show; how does AI help accelerate those positive outcomes,” Ray said. “Or where there’s the malicious bad actor that’s trying [a] frontal attack [on] the platform, how do we block those.”
AI is best when it has thousands of previous examples to learn from, Ray said. The more difficult cases are those outside of the box borrowers, like self-employed applicants, recent immigrants or someone without a credit history or bank account.
Rather than being the decision maker, AI can help prevent discrimination and create a more equal playing field by helping to standardize the process, such as when looking at income, especially from nontraditional sources such as payments through Zelle or Venmo, Ray noted.
It is the type of tool that could have been useful for Navy Federal, which has been accused of discriminatory mortgage lending practices, he said.
Companies can use AI as part of their data analysis, and they may discover things that they didn’t really know before and then build that into a model, Weiss commented, adding “but it wouldn’t be something you would automatically take. You would use the analysis to decide what pieces of decisions should go into the model.”
Regarding underwriting, AI is probably not the biggest thing to be worried about, Weiss said. “It’s also not the thing that’s going to really reduce your costs on an overall basis because you still have to review the file by humans to meet the regulations.”
However, the real potential for benefit for the mortgage industry is in customer service and support, Weiss said.
“That’s going to take some work to really get it right,” he continued. “But I think that there’s an opportunity to provide so much better customer service without the kind of terrible phone trees that people are forced to go through today.”
That is one of the areas where AI can help generate cost savings. But Mann’s point about the system not passing along incorrect information needs to be kept in mind.