Food for AI: Tips for a healthy, high-accuracy database
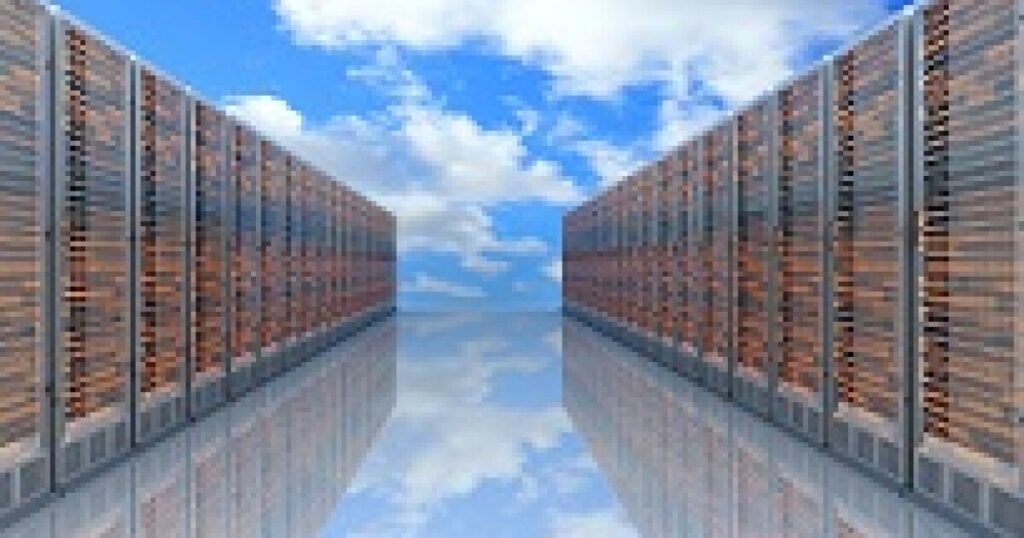
Almost everyone agrees that artificial intelligence (AI) is poised to transform all aspects of an insurance company’s business for the better, but at what cost and effort? The promise of AI, particularly generative AI (Gen AI), lies in its ability to enhance efficiency, accuracy and decision-making capabilities. However, early adoption of these engines has been rife with struggles to train and refine algorithms that often stem from challenges related to the quality, structure and integrity of underlying data.
The data balancing act
A lot of work goes into validating that the underlying database is reliable and consistent. How do you optimize customer information, claims records and other critical data in order to accurately predict risks, identify fraud and streamline claims processing? By mastering the delicate balancing act of feeding AI “brains” the right data without overloading it with too much (and often bad) information — think of that old adage, “garbage in, garbage out.”
Data must be correct, complete and conform to predefined standards or rules. The database must also be considered “high accuracy,” meaning it is free from errors, inconsistencies and duplication. Techniques such as data verification, data cleansing and data quality checks help ensure that the data is accurate and reliable before it is used to train AI models.
In short, what goes in affects what comes out, so it is incumbent upon users to know that the information they are adding to the AI engine is high-quality. It’s easier said than done, but if insurers master a few key principles, they can avoid “hallucinations” and incorrect or even nonsensical outputs.
Structured data is made for AI engines
One of the critical distinctions in AI data management is the difference between structured and unstructured data. Structured data, such as spreadsheets consisting of tables with rows and columns, is organized into a clear and predefined format. These formats are often easier to manage and are easy for AI systems to access, process and analyze.
The good news is that customer information, policy details and claims records are often contained in such spreadsheets or other structured formats. Medical databases, too, usually feature a taxonomy approach that is both specific and general to allow customized searches.
Structured data should be reviewed by both a technical and medical expert, where applicable. All parties should have an eye on consistency in data tagging, labeling, numeric rounding, value advancement, upper-level calculations and formatting.
Standardizing unstructured data
Text documents, images, audio files, videos, handwritten notes, scanned PDFs, emails and random free-text entries in claims forms, on the other hand, are much trickier to sanitize for AI engines. Users must standardize this data as much as possible in order to give it “structure,” so to speak. Give this data a consistent format and set of rules, and it will be easier to compare, analyze and interpret.
Take qualified medical evaluator (QME) impairment rating reports, for example. They should include consistent labeling of body parts, clear definitions of injury types, and uniform calculation methods. Any information on a hand injury should detail relevant data on fingers, the thumb and the wrist, as well as any relevant subcategories. All traumatic brain injury documents could touch on headaches, depression, hearing impairment, vision impairment, dizziness or vertigo, and cognitive impairment.
The same would hold for meeting notes, claims files, adjuster’s reports, and any other category of document. All of them should contain some level of uniformity before being dumped into the data lake.
Ongoing database maintenance
High-accuracy databases are not static; they require regular review to ensure that they continue to meet the needs of AI applications. Ongoing maintenance entails updating data as new information becomes available, reviewing and refining data validation rules, and conducting regular audits to identify and correct any errors or inconsistencies.
In the example of impairment rating, regular maintenance might involve reviewing the coding for different body parts and injury types to ensure that they are still accurate and relevant. It might also entail updating the algorithms used to calculate impairment ratings to reflect new medical guidelines or industry best practices. Or, it could be updating or adding data that reflects clients’ evolving needs to stay ahead of the marketplace.
Without regular maintenance, even the most carefully constructed database can become outdated or corrupted, leading to inaccurate AI outputs.
Transformation depends on what’s underneath
The potential for AI to transform the insurance industry is enormous, but it hinges on the quality and structure of the underlying data, much like how fertile farmland can only reach its full productivity when managed by skilled farmers using the right technology. High-accuracy databases are the fertile soil, but they must be carefully cultivated with structured and standardized data and maintained consistently to yield the best results.
Just as a farmer’s expertise and the application of advanced techniques determine the success of a crop, the effectiveness of AI in the insurance sector depends on a deep understanding of data management. By preparing and optimizing their data, insurers can harness the full power of AI, leading to more reliable, meaningful, and accurate outcomes for their customers and stakeholders.
See more:Gen AI makes data that much more valuable to insurers
Are customers willing to share wellness data with their insurers?