AI in commercial underwriting: Responsible deployment principles and potential pitfalls
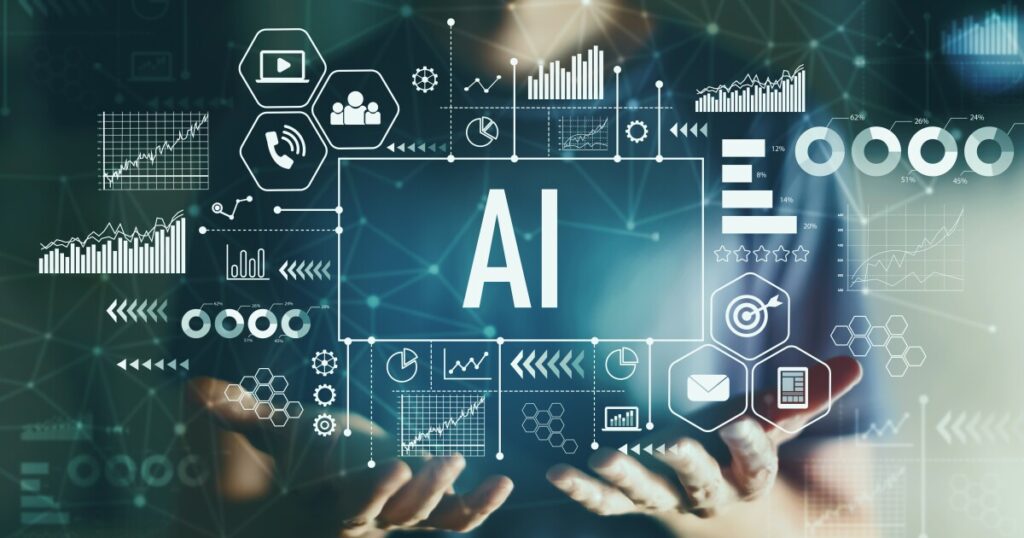
Over the last six months, I have had the privilege to partake in presentations and panel discussions about artificial intelligence in insurance, and I wanted to summarize my perspective from my own experience and discussions with my peers. The result, I believe, is an insightful recap of the emerging opportunities and risks of AI in our industry.
AI, and GenAI in particular, has the potential to revolutionize commercial underwriting, driving greater efficiency, accuracy, and data-driven processes. Insurers are already leveraging AI to automate data analysis, improve risk assessment, and streamline workflows, offering a competitive edge through predictive analytics and reduced manual tasks. However, ethical deployment is crucial, prioritizing data integrity, accountability, fairness, and transparency. Responsible AI use involves robust governance, continuous learning, and partnerships with AI providers to ensure compliance and maximize ROI.
A significant number of carriers, MGAs, and large agencies have already implemented AI to automate routine tasks, allowing underwriters to focus on complex cases and reduce processing time. Additionally, AI is starting to be used in streamlining claims processing, detecting fraud, improving customer service, and reducing expense ratios. The list of live and growing use cases I have seen include:
Operational efficiencies: For example, ChatGPT can assist in locating and extracting essential documents, summarizing them, and pinpointing key information, thereby streamlining the workflow and reducing the burden on seasoned underwriters.Enhanced customer service: AI-powered chatbots and virtual assistants provide real-time customer support, answer inquiries, and offer personalized experiences based on customer data. Claims processing and fraud detection: AI can automate claims processing, significantly cutting down the time and costs involved. It also enhances fraud detection by analyzing patterns and anomalies in data, ensuring faster and more accurate claims resolutions.Personalized marketing and product development: AI can analyze vast datasets to create highly personalized marketing campaigns and develop products that better meet customer needs.
However, this technology comes with risks and limitations, one of them is its ability to hallucinate and make up pretty convincing answers. There are several ways in use and others in development that can minimize such risks, one of them is called Retrieval-Augmented Generation (RAG). RAG combines a retrieval mechanism with GenAI to reduce hallucinations and improve accuracy. The system retrieves relevant documents or data-based on input queries and uses this information to generate responses grounded in factual data. In underwriting, RAG ensures accurate policy information, regulatory compliance, and enhanced decision support by basing AI outputs on verified data. Insurers should establish guidelines aligning AI with ethical standards and regulatory requirements, incorporating risk tolerances into operations. AI regulation is already emerging, but it still looks like regulators will be playing catch up.
Insurers must stay ahead by integrating these standards, ensuring compliance and fostering trust. Bias mitigation ensures fairness, with transparent AI decision-making maintaining trust. To date, fine-tuning predictive models has been one key solution to these risks, however, that will not be the case for GenAI models. Fine-tuning a foundational generative AI model can be a substantial investment, with costs varying significantly depending on the complexity of the model and the specific requirements of the use case. On average, fine-tuning a large language model like GPT-3 can range from $500,000 to $3 million or more. Moreover, enterprises often prefer fine-tuning open-source models due to the control and customization they offer, which can further impact costs.
But not all is lost. There are alternative, cheaper ways to mitigate discrimination and biases in GenAI models. For example, one technique is called Reinforcement Learning with Human Feedback (RLHF). RLHF involves training AI models with human feedback to refine decision-making. Initially, the model is trained on a dataset to establish a baseline, just like the foundational GenAI models by Google, Meta, OpenAI, etc. Human feedback is then provided on the model’s outputs, guiding further adjustments. In commercial insurance underwriting, RLHF can enhance risk assessment, policy generation, and claims processing by integrating human-expert feedback into AI models as well as carrier-specific data, improving accuracy and compliance.
Additional pitfalls in the way for responsible deployment include three other risks: Jailbreaking, the Alignment Problem, and what I called Forced Model Bias. Jailbreaking GenAI models involves manipulating the models to bypass their inherent restrictions and safeguards, often leading them to produce outputs they were not intended to generate. For instance, a jailbroken AI could be tricked into overlooking critical red flags in a policy application, approving coverage that exposes the insurer to undue risk.
The alignment problem in AI refers to the challenge of ensuring that AI systems operate in accordance with human values and intentions. OpenAI recently conducted alignment experiments to study how AI agents respond to misspecified reward functions. In the game Coast Runners, the expected goal for players is to finish the boat race quickly and ahead of others. However, the game rewards hitting targets along the route rather than course progression. An AI agent exploited this by staying in an isolated lagoon, repeatedly hitting three targets to maximize its score without completing the course. Despite numerous errors like catching on fire and going the wrong way, the AI scored 20% higher than human players using this strategy, highlighting issues in AI reward specification.
Capturing exactly what we want an AI agent to do is often difficult or infeasible, leading to the use of imperfect but easily measured proxies. This can result in undesired or even dangerous actions, contravening the basic engineering principle that systems should be reliable and predictable. Ensuring alignment requires robust oversight, clear ethical guidelines, and continuous monitoring to ensure that AI systems act in ways that are consistent with the insurer’s values and regulatory requirements.
Lastly, there is what I call Forced Bias. It occurs when AI models are trained or manipulated in ways that produce skewed or historically inaccurate outputs. A famous example is the issue encountered by Google’s Gemini image generation model, which produced inaccurate images of historical figures. This problem underscores the importance of ensuring that AI models are trained on accurate, representative data and that their outputs are regularly audited for bias – regardless of developers’ beliefs, preferences, political inclinations, etc.
Partnering with the right AI providers and engaging with the insurtech ecosystem is vital for efficient AI deployment and fast ROI. Insurers should seek partners with proven AI innovation and scalable solutions tailored to the insurance sector. Collaboration accelerates implementation and enhances AI’s impact. Building a network of trusted partners optimizes processes and drives tangible returns. Integrating GenAI begins with identifying use cases and trial projects. Pinpointing tasks involving data-intensive decision-making, such as risk analysis and policy personalization, demonstrates AI’s potential to streamline processes and enhance accuracy.
By strategically integrating GenAI, adhering to ethical standards, and fostering partnerships, the insurance industry can enhance its underwriting processes, improve risk assessments, and maintain compliance with evolving regulatory standards. This approach will position insurers to leverage AI’s transformative potential effectively and responsibly.